Deploy Use-Case Specific Apps
Apps built for specific requirements of every organization across industry vertical
- BioPharma
- Pharma
- Chemicals
- Oil & Gas
.png?width=400&height=311&name=Batch%20alarm%20analysis%20and%20interpretation%20(2).png)
Bioreactor Scale Up
Bioreactor scale-up is the process of moving from a small-scale bioreactor used in research to a larger one for commercial production. This step is crucial for producing biopharmaceuticals like vaccines and enzymes. Bioreactors are controlled vessels for growing cells or microorganisms. Efficient scale-up ensures consistent product quality and yield.
.png?width=400&height=311&name=Batch%20alarm%20analysis%20and%20interpretation%20(2).png)

- Process control: Monitoring and controlling things like pH, temperature, and nutrients is more complex in larger systems.
- Shear stress: Higher agitation speeds in big reactors can harm delicate cells.
- Heat transfer: Removing heat efficiently is harder in larger volumes, but it's needed for optimal cell growth.

- Computational modeling: Software tools predict how parameters like mixing and mass transfer behave in larger bioreactors, helping optimize design and operation.
- Pilot-scale studies: Medium-sized bioreactors (pilot plants) test and refine the process before full-scale production.
- Scalable bioreactor design: Bioreactors with better mixing and mass transfer, like those with special impellers or internal baffling, are used.
- Single-use bioreactors: Disposable bioreactors reduce contamination risk and speed up processes, making scale-up easier.

- Consistent product quality : Optimal growth conditions ensure consistent product quality and effectiveness.
- Increased production capacity : Bigger bioreactors produce more to meet market needs.
- Shorter time to market : Faster scale-up speeds up delivery of biopharmaceuticals to patients.
- Cost-effective production : Large-scale production reduces the cost per unit.
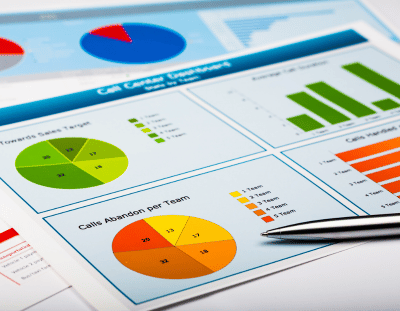
Lyophilization
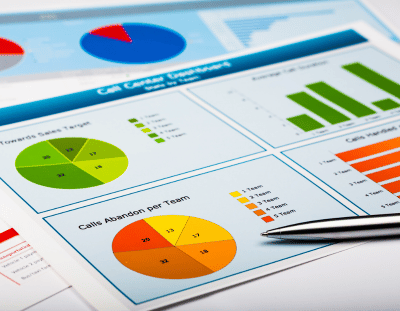

- Product Stability: Not all products respond well to freezing and dehydration. Some may lose potency or suffer structural damage.
- Uniform Drying: Ensuring even drying throughout the product is crucial to maintain quality, which can be difficult for complex shapes or large volumes.

- Process Optimization: Advanced lyophilization cycles with tailored temperature and pressure profiles can improve drying efficiency and product stability.
- Formulation Optimization: Adding specific ingredients, called bulking agents, can help maintain product structure during drying.

- Improved Stability: Lyophilized products are more resistant to degradation from heat, light, and moisture.
- Enhanced Delivery: Freeze-drying can improve the reconstitution or dispersion properties of a product, making it easier to administer or use.
- Extended Shelf Life: Removing water prevents microbial growth and spoilage, significantly extending the product's shelf life.
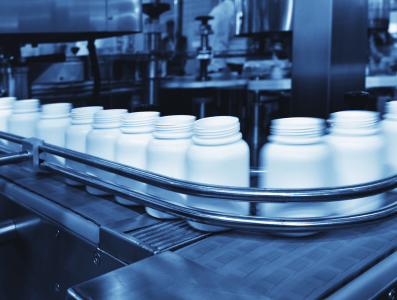
Fill-finish
Fill-finish is the final stage of aseptic (germ-free) manufacturing, where a sterilized drug product is filled into vials, syringes, or other containers and sealed. This critical step ensures the product's sterility, purity, and dosage accuracy for safe and effective use.
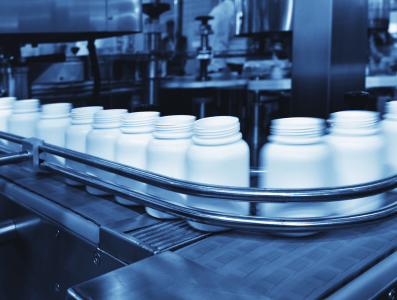

- Product Properties: Some drugs are sensitive to factors like temperature, light, or agitation during filling, requiring careful handling.
- Filling Accuracy: Precise and consistent filling is crucial to ensure patients receive the correct dosage.

- Process Validation: Rigorous testing and validation procedures ensure the effectiveness of the fill-finish process for each specific product.
- Container Selection: Choosing the right container material and closure system helps ensure product compatibility and sterility.

- Scalability: Modern fill-finish processes can be adapted to handle production of small or large batches efficiently.
- Extended Shelf Life: Proper aseptic filling protects the product from degradation, extending its shelf life.
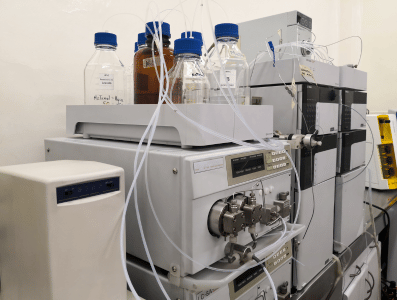
Chromatography
In Chromatography, complex mixtures are separated based on the interaction of molecules with a stationary and mobile phase. This allows scientists to isolate and purify desired biopharmaceutical products, such as proteins and antibodies, from cell culture broths containing a variety of cellular components and impurities.
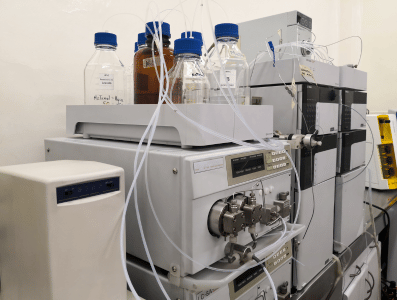

- Scalability: Transferring a well-functioning chromatography process from small-scale research to large-scale production requires careful optimization.
- Cost-effectiveness: Chromatography resins and processes can be expensive and optimizing them for high yield and purity is crucial.

- Process Optimization: Through careful optimization of factors like flow rates, buffer conditions, and column size, scientists can achieve efficient and cost-effective purification.
- Resin Selection and Development: Choosing the right chromatography resin with specific binding properties is crucial for efficient purification. Additionally, some companies develop custom resins tailored to specific biopharmaceutical products.

- Process Efficiency: Optimized chromatography processes minimize waste and production costs.
- Improved Consistency: Consistent product quality is crucial for regulatory approval and patient safety.
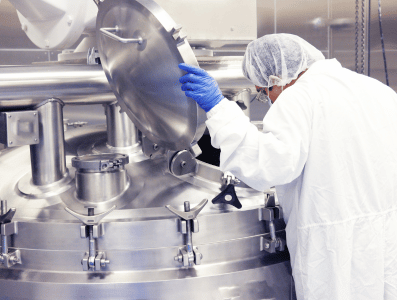
Bioreactor Monitoring
In Bioreactor monitoring, there is a continuous measurement and analysis of critical parameters within a bioreactor during cell culture processes for biopharmaceutical production. These parameters influence cell growth, product yield, and overall process success. Effective monitoring allows for real-time adjustments and ensures optimal conditions for product development.
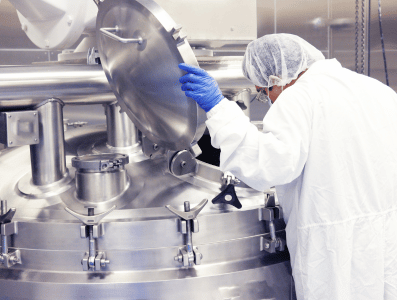

- Data Interpretation: Interpreting the complex interplay of various parameters requires expertise and advanced analytical tools.
- Multiple Parameters: Monitoring a wide range of parameters like pH, temperature, dissolved oxygen (DO), and nutrient levels is crucial.

- Multivariate Analysis Tools: Advanced software tools analyze complex data sets, identifying trends and potential issues before they impact product yield.
- Automation and Control Systems: Automated systems collect and analyze data, enabling real-time adjustments to maintain optimal growth conditions.

- Process Optimization: Data analysis allows for process optimization, leading to increased product yield and reduced production costs.
- Improved Process Control: Real-time data allows for adjustments to maintain optimal cell growth and product formation.
- Enhanced Product Quality: Precise control of bioreactor conditions minimizes variability and ensures consistent product quality.
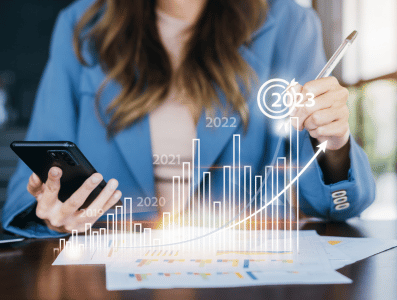
Titer predictions
Predicting titer accurately is crucial for optimizing bioprocesses and maximizing production yield. By knowing the expected titer beforehand, manufacturers can make informed decisions about resource allocation, downstream processing needs, and potential bottlenecks.
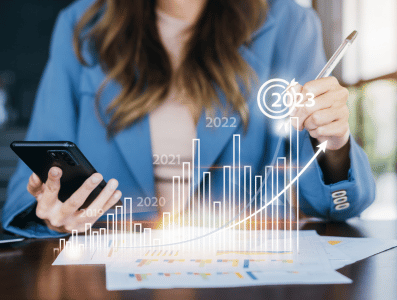

- Limited Data: Early-stage bioprocesses may have limited historical data, making it difficult to build robust predictive models.
- Process Complexity: Numerous bioprocess parameters, including temperature, pH, and agitation, influence cell growth and product formation.
- Biological Variability: Cell cultures are inherently variable, with factors like cell line stability and nutrient availability impacting product yield.

- Physics-Based Modeling: Simulate the biological processes within the bioreactor can be used to predict the impact of different conditions on titer.
- Real-Time Monitoring: It helps in monitoring of various bioreactor parameters, along with cell health indicators, and can provide valuable data for more accurate titer predictions.
- Machine Learning (ML): ML algorithms can analyze large datasets of historical bioprocess data, identifying patterns and relationships between process parameters and resulting titer.

- Process Optimization: Knowing the expected titer allows for adjustments to bioprocess parameters to maximize product yield.
- Reduced Costs: Accurate predictions can help minimize wasted resources and optimize downstream processing based on the anticipated product concentration.
- Informed Decision-Making: Titer forecasts enable better planning for production scheduling and resource allocation.
- Faster Development Time: Early and accurate titer predictions can help accelerate biopharmaceutical development timelines.
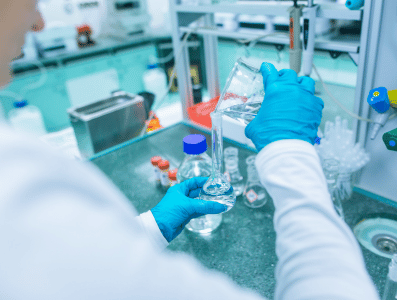
Process Scale-Up
In the Pharma industry, process scale-up refers to transitioning a drug's production from small laboratory batches to large-scale commercial manufacturing. To meet market demands the process parameters need to be maintained for safety, quality, and efficacy.
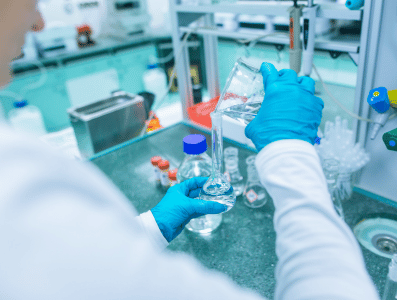

- Maintaining Product Consistency: Scaling up can alter factors like mixing, heating, and cooling, potentially affecting the drug's properties. Ensuring the final product has the same characteristics as the lab-scale version requires careful process optimization.
- Equipment Limitations: Laboratory equipment may not be suitable for large-scale production. Identifying appropriate equipment with sufficient capacity and addressing potential limitations during scale-up is crucial.

- Modeling and Simulation: Computational modeling and simulation tools can help predict how process changes will affect product quality, allowing for proactive optimization.
- Pilot-Scale Production: Intermediate-sized pilot batches bridge the gap between lab and commercial scale. Data from pilot production helps identify and address scalability challenges before full-scale manufacturing.
- Process Understanding: Thorough understanding of the underlying chemistry and physics of the manufacturing process is critical for predicting and mitigating potential issues during scale-up.

- Efficient Manufacturing: A well-designed scale-up process leads to efficient production with minimal waste and downtime.
- Consistent Product Quality: Scalability studies ensure the final drug product meets all quality and safety requirements.
- Faster Time to Market: Efficient scale-up reduces delays and allows drugs to reach markets sooner.
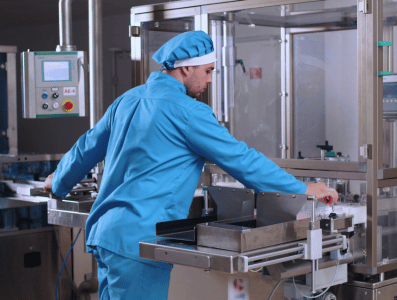
Process Optimization
Process optimization involves continually improving the pharmaceutical manufacturing process to enhance efficiency, quality, and cost-effectiveness. This ensures consistent production of high-quality drugs while minimizing waste and production time.
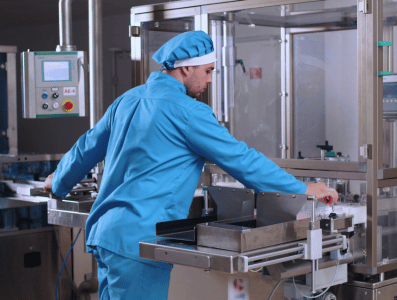

- Efficiency Bottlenecks: Identifying and eliminating bottlenecks that slow down production is crucial for process optimization. These bottlenecks can occur at various stages, from raw material handling to final packaging.
- Yield Variability: Inconsistencies in the manufacturing process can lead to variations in product yield. Optimizing the process minimizes such variations and ensures consistent production of usable drug products.

- Data Analytics: Utilizing data from various sources, including production logs, equipment sensors, and quality control testing, allows for identifying areas for improvement and optimizing process parameters.
- Continuous Improvement Culture: Fostering a culture of continuous improvement encourages all personnel to identify and suggest ways to optimize the process, leading to ongoing advancements.

- Reduced Costs: Process optimization can significantly reduce manufacturing costs by minimizing waste, optimizing resource utilization, and streamlining operations.
- Improved Product Quality: Consistent process control minimizes variability and ensures consistent production of high-quality drugs.
- Faster Time to Market: By enhancing efficiency, optimized processes can reduce production timelines and expedite drug availability to patients.
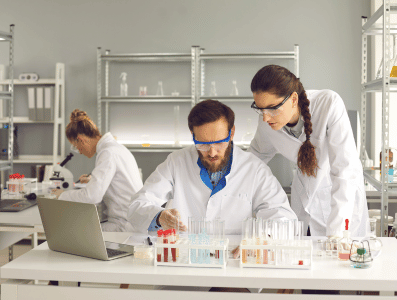
Development and Review of Process Design
Developing and reviewing process design is a critical stage in bringing a new drug to market. It involves creating a well-defined, efficient, and scalable manufacturing process that ensures consistent production of a high-quality drug product. It requires careful consideration of various factors like raw materials, equipment capabilities, regulatory requirements, and safety.
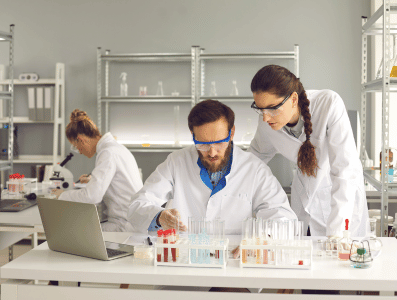

- Balancing Efficiency and Quality: Designing a process that is both efficient (cost-effective and timely) and ensures consistent production of a high-quality drug can be challenging. Trade-offs may be necessary, and careful optimization is crucial.
- Scalability: The initial process design needs to be adaptable for future scale-up to commercial production volumes. This requires considering factors like equipment limitations and potential changes in process behavior at larger scales.

- Process Simulation: Utilizing computer modeling tools can help predict how different process parameters will affect product quality and performance. This allows for virtual optimization before real-world implementation.
- Quality by Design (QbD): This approach emphasizes building quality into the design stage itself. QbD involves understanding the critical factors that affect product quality and designing a process that controls these factors.

- Robust and Efficient Manufacturing Process: A well-designed and reviewed process is reliable, efficient, and cost-effective for producing high-quality drugs.
- Smoother Scale-Up: Designing for scalability allows for a smoother transition from lab-scale development to large-scale commercial manufacturing.
- Faster Time to Market: Efficient process design and review contribute to a faster development timeline, bringing new drugs to patients sooner.
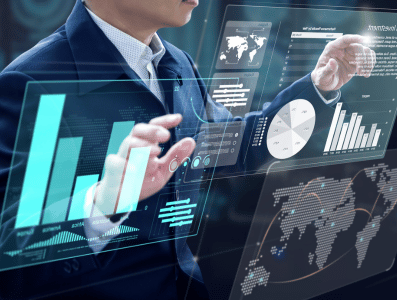
Data Analysis
Data can come from diverse sources and effective data extraction allows researchers, developers, and other stakeholders to analyze vast amounts of information and gain valuable insights to drive progress.
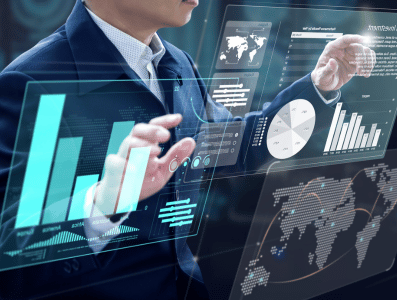

- Data Silos: Pharmaceutical companies often have data scattered across different departments and systems. This fragmentation makes it challenging to extract and integrate data for comprehensive analysis.

- Data Integration Platforms: These platforms can connect to disparate data sources, extract relevant information, and consolidate it into a central repository. This facilitates consistent data access and analysis.
- Standardization and Normalization: Implementing data standards and normalization techniques ensures data from different sources is consistent and readily usable for analysis.

- Improved Decision Making: Extracted and analyzed data can provide valuable insights for informed decision-making across various aspects of the pharmaceutical industry, such as drug discovery, clinical trial design, and marketing strategies.
- Optimized Manufacturing Processes: Process data extraction allows for monitoring and optimizing production processes, leading to improved efficiency, cost reduction, and reduced waste.
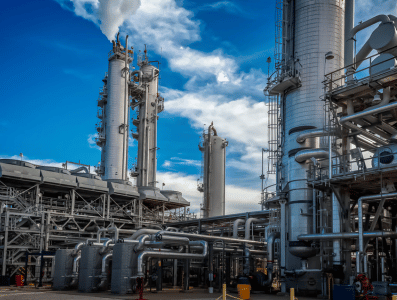
Process Optimization
Process optimization involves continuously evaluating and improving chemical production processes. The goal is to maximize efficiency, safety, profitability, and environmental sustainability. This can involve fine-tuning existing processes or implementing new ones.
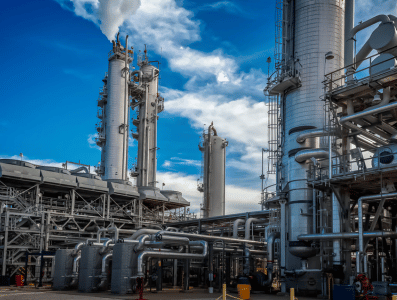

- Process Complexity: There are multiple process parameters that are involved in a process. Identifying areas for improvement and implementing changes without compromising safety or product quality requires careful analysis.

- Data Analytics and Modeling: Leveraging process data, production logs, and sensor information allows for identifying bottlenecks, inefficiencies, and opportunities for improvement. Machine learning and process modeling tools can help predict the impact of process changes before implementation.

- Increased Efficiency and Productivity: Optimized processes lead to higher production rates, reduced waste generation, and lower operating costs.
- Improved Product Quality: Consistent process control minimizes variability and ensures consistent production of high-quality chemicals that meet customer specifications.
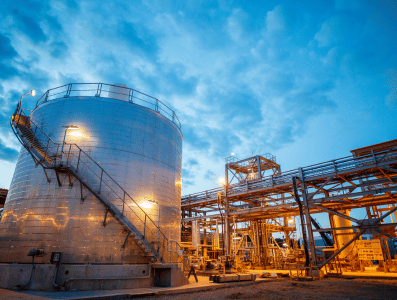
Pipe Erosion
Pipe erosion occurs when chemicals flowing through pipes wear away the pipe walls due to mechanical or chemical attack. This leads to pipe thinning, leaks, and potential catastrophic failures. Erosion can significantly impact safety, production efficiency, and overall operational costs.
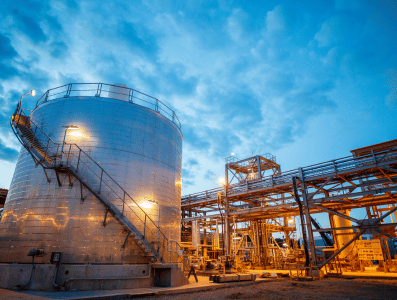

- Multiphase Flow: Chemical processes often involve multiphase flows, where liquids, solids, and gases flow together through pipes. Solid particles can cause significant abrasive wear, while gas bubbles can accelerate erosion by creating turbulent flow patterns.
- Predicting Erosion Rates: Accurately predicting erosion rates for different chemicals and flow conditions is complex. This makes it challenging to select appropriate pipe materials and thicknesses for optimal performance and lifespan.

- Process Optimization: Adjusting process parameters like flow velocity and temperature can reduce erosive forces. Additionally, removing solid particles from the flow stream through filtration or settling tanks can minimize abrasive wear.
- Erosion Monitoring and Inspection: Regularly monitoring pipe wall thickness with ultrasonic testing or other techniques allows for early detection of erosion and timely maintenance or replacement.

- Enhanced Safety: Mitigating pipe erosion minimizes the risk of leaks and catastrophic failures, ensuring safer plant operations and protecting personnel and the environment.
- Improved Uptime and Efficiency: Reduced pipe failures and proactive maintenance lead to less downtime and improved overall production efficiency.
- Reduced Maintenance Costs: Early detection and prevention of erosion minimizes the need for emergency repairs and replacements, leading to lower maintenance costs in the long run.
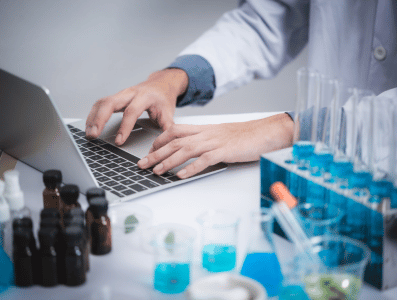
Data Analysis
Data can come from diverse sources and effective data extraction allows researchers, developers, and other stakeholders to analyze vast amounts of information and gain valuable insights to drive progress.
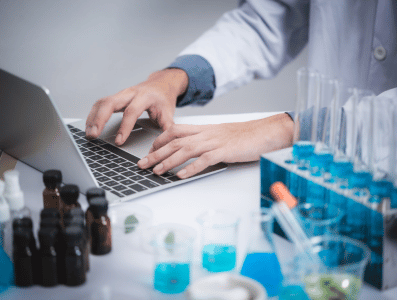

- Data Silos: companies often have data scattered across different departments and systems. This fragmentation makes it challenging to extract and integrate data for comprehensive analysis.

- Data Integration Platforms: These platforms can connect to disparate data sources, extract relevant information, and consolidate it into a central repository. This facilitates consistent data access and analysis.
- Standardization and Normalization: Implementing data standards and normalization techniques ensures data from different sources is consistent and readily usable for analysis.

- Improved Decision Making: Extracted and analyzed data can provide valuable insights for informed decision-making across various aspects
- Optimized Manufacturing Processes: Process data extraction allows for monitoring and optimizing production processes, leading to improved efficiency, cost reduction, and reduced waste.
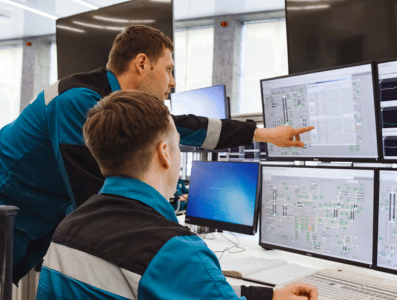
Erosion Monitoring
Internal erosion within pipes, valves, and other equipment caused by produced fluids carrying sand, gas, and other abrasive particles. Erosion can lead to equipment failure, production loss, safety hazards, and costly repairs. Monitoring helps detect erosion early for timely intervention.
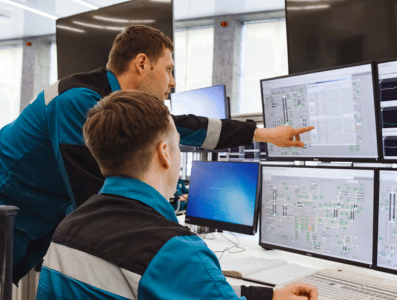

- Multi-factorial issue: Erosion rates depend on various factors like fluid properties (flow rate, pressure, sand content), equipment design, and material properties.
- Need real-time data: Traditional inspection methods involve shutdowns for physical measurements, leading to production loss. The industry needs real-time or continuous monitoring solutions.

- Computational Modeling: Software models can be used to predict erosion rates based on operational data (flow rates, pressures) and material properties. This helps identify high-risk areas.

- Reduced downtime: Early detection of erosion allows for preventative maintenance and repairs before equipment failure occurs, minimizing production downtime.
- Optimized maintenance: Data from monitoring systems helps target maintenance efforts on critical equipment, optimizing resource allocation.
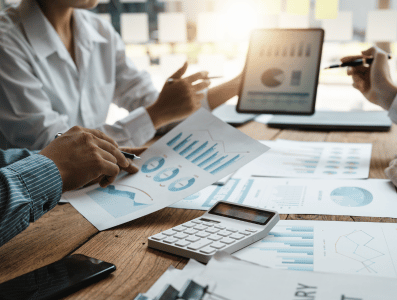
Data Analysis
Large amounts of data are generated from exploration and drilling to production, transportation, and refining. Data analysis transforms this raw data into actionable insights that drive better decision-making across all aspects of the business.
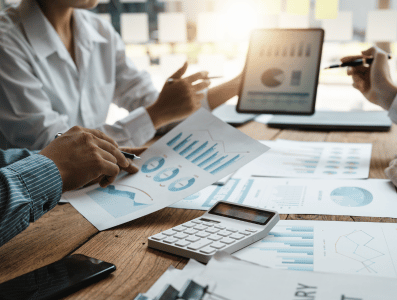

- Data Silos: Information often resides in isolated systems across different departments, making it difficult to obtain a holistic view of operations.
- Data Quality: Data inconsistencies, errors, and missing values can hinder analysis and lead to misleading conclusions.
- Complexity of Data: Oil & Gas industry deals with diverse data types, from geological formations to sensor readings and financial records. Integrating and analyzing such complex data sets requires advanced tools and expertise.

- Advanced Analytics Techniques: Machine learning, artificial intelligence (AI), and big data analytics unlock hidden patterns and insights from complex datasets. Predictive analytics can forecast equipment failures, optimize production, and identify new exploration opportunities.
- Data Integration Platforms: These platforms consolidate data from various sources into a central repository, enabling unified access and analysis.
- Data Cleaning and Standardization: Techniques like data cleansing and transformation ensure data accuracy and consistency for reliable analysis.

- Improved Exploration & Production Efficiency: Data analysis helps optimize drilling locations, predict reservoir characteristics, and improve well production.
- Enhanced Safety and Risk Management: Real-time sensor data analysis can predict equipment failures and prevent safety incidents.
- Cost Optimization: Data-driven insights can help identify areas for cost reduction across the entire value chain, from optimizing drilling operations to streamlining logistics.
- Data-Driven Decision Making: Leaders can make informed choices based on real-time insights instead of relying on intuition or experience alone.
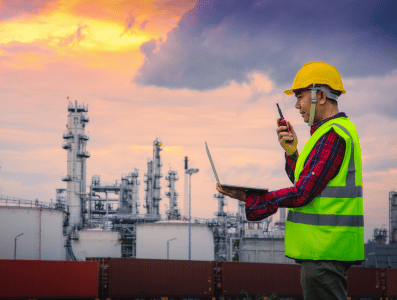
Computational Complexity
The oil and gas industry involves complex systems which involve a vast amount of data, complex physics, and numerous interacting factors. Understanding and optimizing these systems requires significant computational power. This inherent complexity creates challenges.
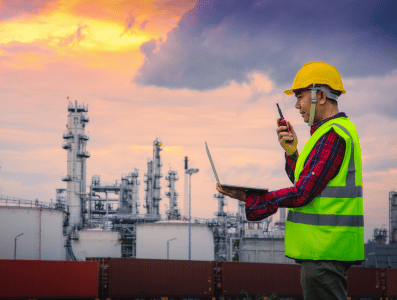

- Multi-Physics Simulations: Accurately modeling oil and gas reservoirs involves simulating fluid flow, rock mechanics, and thermal properties. These simulations require complex algorithms and high computational resources.
- Decision-Making Under Uncertainty: Subsurface geology is inherently uncertain. Exploration and production decisions require evaluating various scenarios with incomplete information, which adds a layer of computational complexity.
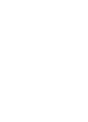
- Machine Learning (ML): Machine learning can be used to identify patterns in complex data, automate workflows, and improve decision-making, potentially leading to reduced computational demands.
- High-Performance Computing (HPC): Utilizing powerful computer clusters with parallel processing capabilities allows for faster and more efficient data analysis and simulation.

- Improved Efficiency: By overcoming computational complexity, companies can optimize reservoir management, reduce drilling costs, and improve production efficiency.
- Better Decision-Making: Advanced computational tools can provide more accurate simulations and data analysis, leading to better-informed decisions throughout the oil and gas value chain.